Contents
Purpose of the Project
Data anonymization is required before a big-data business can run effectively without compromising the privacy of personal information it uses. It is not trivial to choose the best algorithm to anonymize some given data securely for a given purpose. In accurately assessing the risk of data being compromised, there needs to be a balance between utility and security. Therefore, using common pseudo microdata, we propose a competition for the best anonymization and re-identification algorithm.
The project addresses the aim of the competition, the target microdata, sample algorithms, utility and security metrics. The design of an evaluation platform is also studied.
De-identified information
In Japan, the Personal Information Protection Commission (PPC) has put into fully effect the amended Act on the Protection of Personal Information on May 30, 2017. The notion of “Anonymously Processed Information (API)” has introduced as a sort of de-identified information that satisfies two conditions, processed to be unidentifiable to said person, and prohibited from restoring said personal information. However, the enforcement rules has some uncertainty in declaring data to be API (see the table of rules).
rule | description | example |
---|---|---|
(1) | Deleting a whole or part of descriptions which can identify a specific individual | Name, address, date of birth, telephone number |
(2) | deleting all individual identification codes | passport number, driver’s license, biometric (DNA, face) |
(3) | deleting codes linking mutually plural information | management IDs, email address |
(4) | deleting idiosyncratic descriptions etc. | medical history (# cases is small), “116 years old” |
(5) | taking appropriate action based on the other measures on attribute etc. of PI database | records/cell suppression, generalization, top-coding, service IDs, purchase history, transpiration history |
figure: Enforcement Rules
Competition Design
To address the issues in anonymization of big data, we propose an open style data competition. We focus on “records re-identification” risk and defines baseline utility functions and some re-identification algorithms. With arbitrary techniques, the best anonymization dataset is determined. Table shows the PWS Cup editions held so far, as a part of academic conference, IPSJ computer security symposiums (CSS) since 2015.
2015 | 2016 | 2017 | |
---|---|---|---|
Date Venue | 10/21-22 Nagasaki Brick H. | 10/11-12 Akita Castel H. | 10/23-24 Yamagata Int. H. |
Participants | 13 teams 20 participants | 15 teams 42 participants | 14 teams 43 participants |
Dataset | NSTAC Synthesized data | UCI Dataset “Online Retail” POS records | |
# att. | 25 | 11 (customer 4 att. + transaction 7 att.) | |
# persons | 8,333 | 400 | 500 |
# records | N/A | 18,524 | 44,917 |
duration | 1 year | 1 year | 12 months |
![]() | ![]() | ![]() |
figure: PWS Cup Competition seriese
Result and Future
Figures illustrate how our competition evaluates the utility of de-identified data and the risk to be reidentified. Based on pre-defined utility functions, we automate the process of risk evaluation and found the tradeoff between utility and privacy in data anonymization. We will explore reasonable and reliable schemes for the technologies.
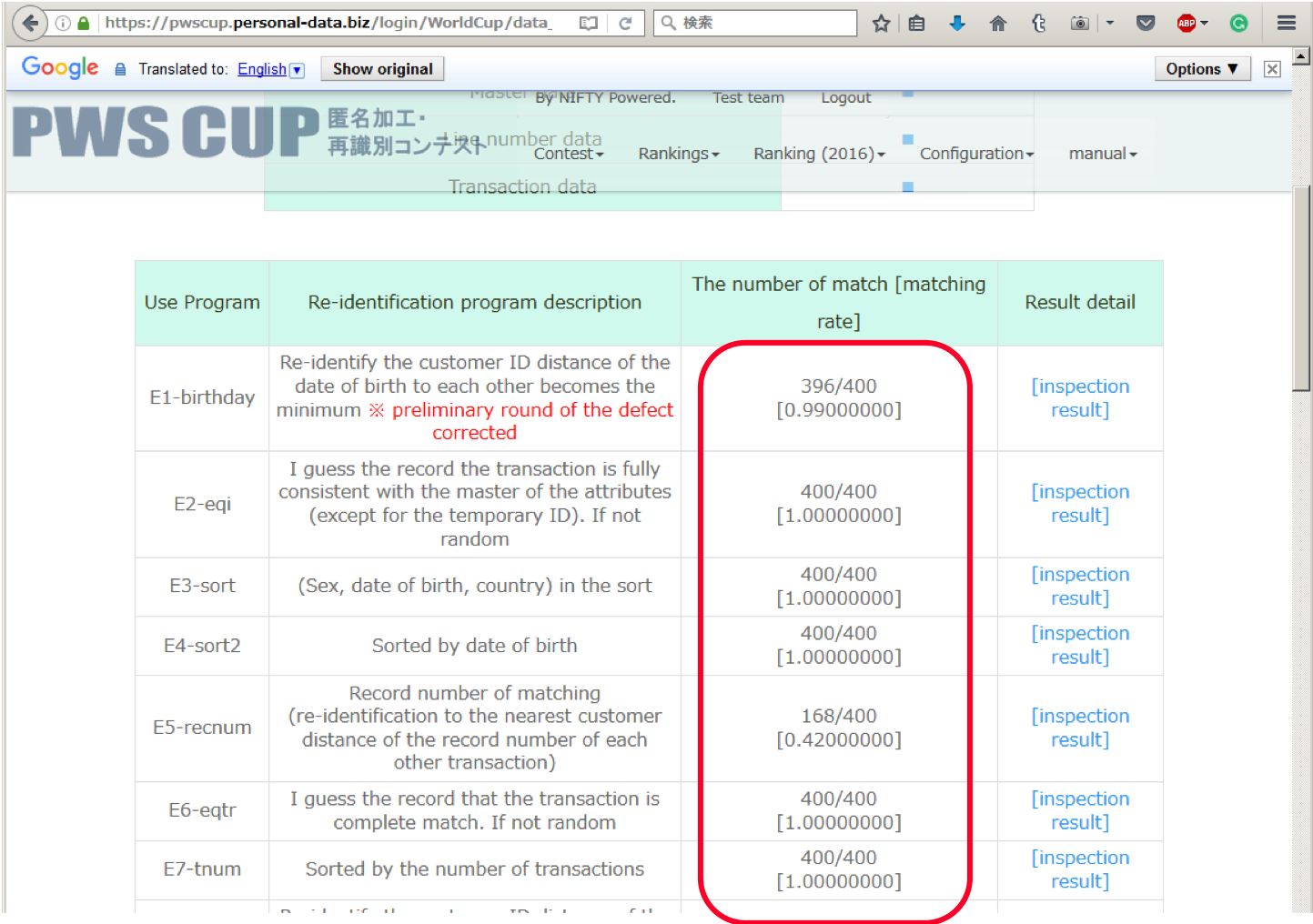
figure: Automate Risk Evalutation
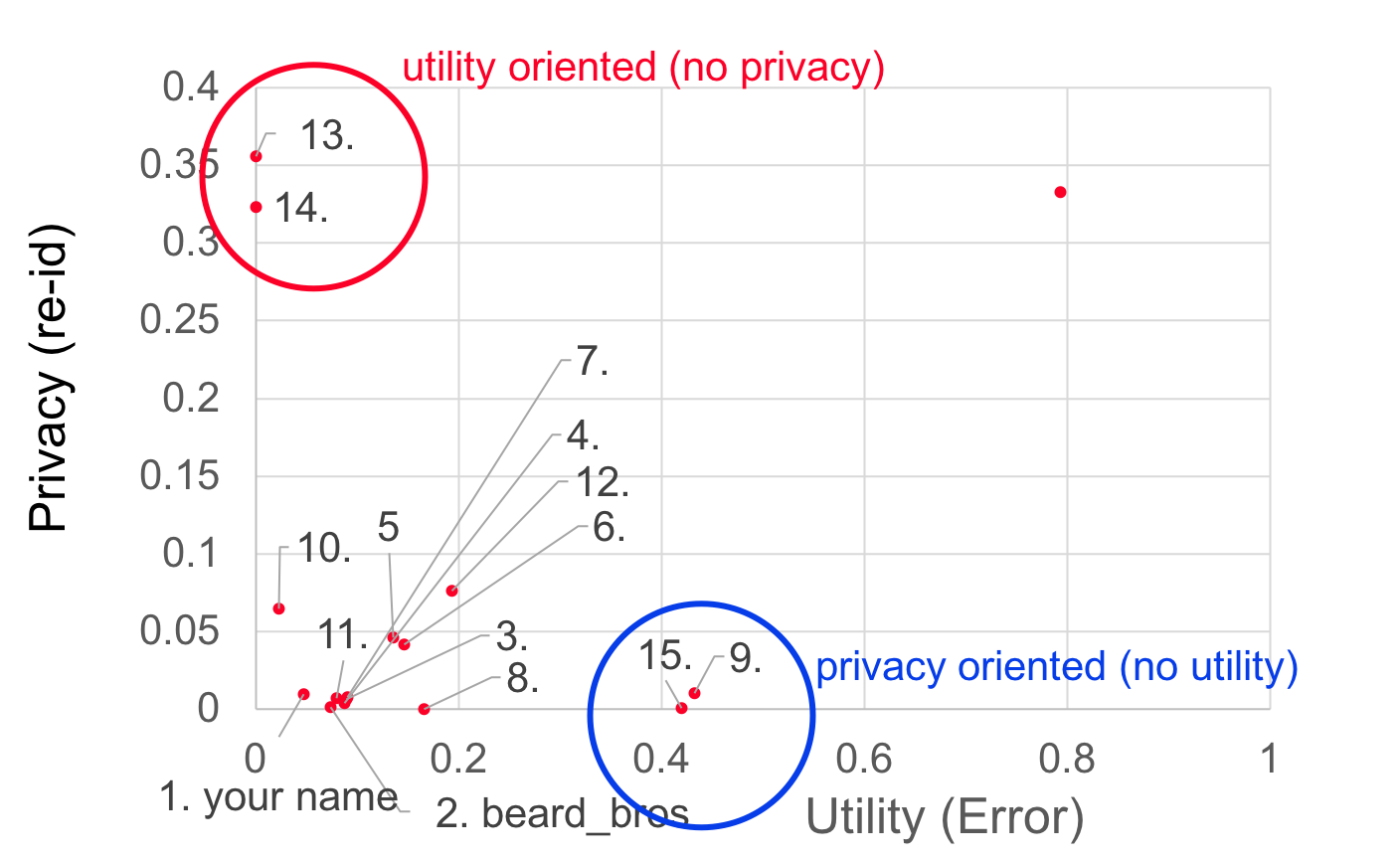
figure: Utility-Privacy Tradeoff